FaCeT
Understanding Uncertainty
One of the biggest challenges to understanding, projecting, and managing the impact of climate change on fisheries is accounting for and communicating uncertainty. We use uncertainty to measure our confidence in the validity of a finding based on the type, amount, quality, consistency, and agreement of evidence (sensu Intergovernmental Panel on Climate Change, 2010).
Uncertainty in climate projections is not something to fix or solve. Rather, uncertainty is inherent to our understanding of ocean systems and their response to climate change. Yet, different strategies and perspectives provide insight and information into the factors that influence uncertainty and how to account for it so that proactive approaches to climate readiness and resilience can be developed.
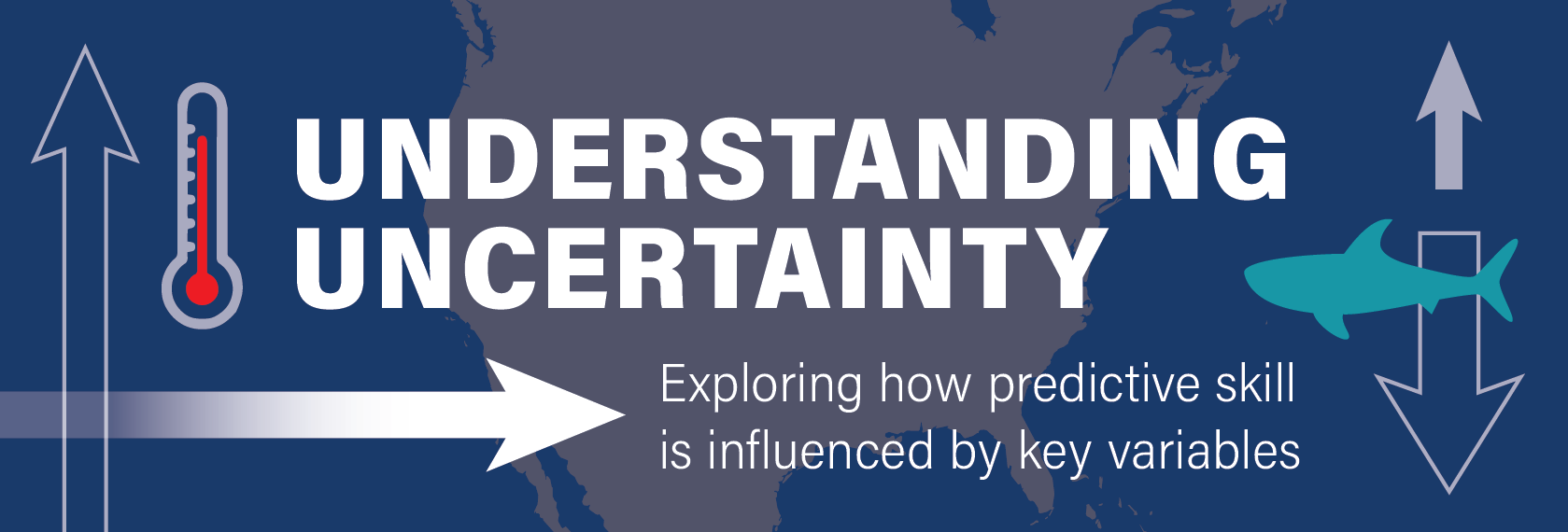
Here, we explore and discuss three sources of uncertainty in projecting climate impacts on fisheries:
- differences among global climate models
- extrapolating to novel ocean conditions
- imperfect ocean sampling
For each source, we review approaches that can be used to incorporate and account for uncertainty. For a more detailed exploration of the relative influence of different sources of uncertainty on climate impact projections, see Brodie et al. (2022).
Differences among global climate models
To understand the range of potential impacts of climate change on fisheries, scientists typically use species data paired with models that project future oceanographic conditions. Because climate models differ, each model yields different projections of how species will be impacted by climate change over time.
For example, the three colored lines in the following figure show differences in the projections of three different climate models that span from 1980-2100. These models predict how habitat suitability will likely change due to climate change for swordfish and shortfin mako, two key fishery species in the California Current System (CCS).
The colored lines compare the projections of the climate models from the Geophysical Fluid Dynamics Laboratory (GFDL) in red, Hadley Center (HAD) in green, and Institut Pierre Simon Laplace (IPSL) in blue. The dashed black line shows the mean of the three models.
Due to variability among climate models, results from the three models exhibit differences in their predictions of how habitat for these two species will change over time. These differences also reflect the species’ ecological characteristics and their ability to adapt to changing environmental conditions.
Extrapolating to novel ocean conditions
A second source of uncertainty in model predictions arises when models extrapolate beyond the bounds of the historical, observed range of ocean conditions. A measure of how different or how novel ocean conditions are can be captured to quantify the degree to which future ocean conditions extend beyond historical ranges. This measure, known as the degree of novelty metric, varies across ocean areas based on their underlying dynamics.
For example, the CCS and Northeast Shelf Large Marine Ecosystem (NES) have both experienced climate-driven warming in recent years. However, the CCS ecosystem sea surface temperatures are more episodic, with relatively high year-to-year variability compared to the NES. In turn, the degree of novelty of recent temperature conditions is higher in the NES as rising average temperatures more readily fall outside the range of historically observed conditions.
One effective strategy to address this inherent uncertainty is to use model averaging or ensembling, which combines predictions from multiple models. This approach has been demonstrated as a robust and effective way to overcome biases inherent in individual models.
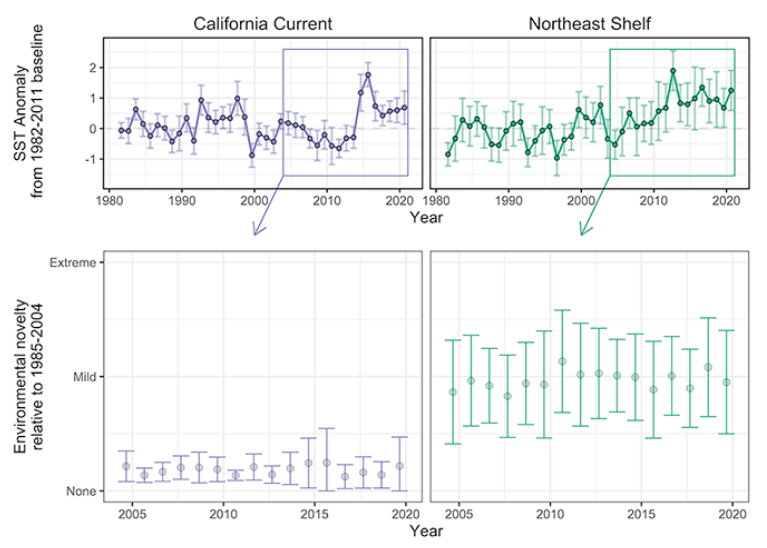
What we found
To understand how uncertainty can arise from novel ocean conditions, we used a simulation framework to consider how the predictive skill of species distribution models changed as a function of the novelty of the oceanographic conditions. A simulation model allows us to interrogate specific sources of uncertainty and better understand their influence on a known, predetermined outcome.
We found that prediction skill declines as the degree of environmental novelty increases. This was consistent across both the CCS and NES large marine ecosystems, despite their different underlying ocean dynamics. In ecosystems like the NES, which is experiencing conditions that are further outside historical, observed ranges, we should therefore expect to have less certainty or confidence in climate impact projections.
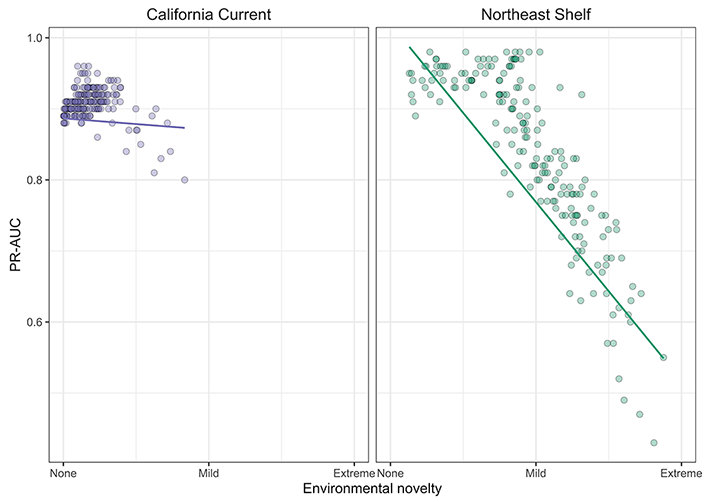
Because species distribution models continue to be a powerful tool to track climate change impacts, these results highlight the importance of understanding how novel environmental conditions influence model uncertainty. This is particularly important for ecosystems like the NES, where conditions are relatively novel.
Imperfect ocean sampling
A third source of uncertainty in predictive modeling arises from imperfect sampling of the ocean. Data collected for marine species is often categorized as fishery-independent or fishery-dependent, given significant differences in how these data types represent species’ occurrence.
Examples of fishery-independent data include aerial or shipboard transects or electronic telemetry tags that track an individual animal’s movement. Fishery-dependent data, in contrast, come from fishing activity and would include data from captain logbooks or an on-board observer program. Fishery-dependent and -independent data types are all characterized by different strengths and limitations.
What we found
We compared models built with four different types of species occurrence data for the blue shark, a heavily-exploited pelagic fish species, to understand how the different types of data can influence the predictive skill of model projections.
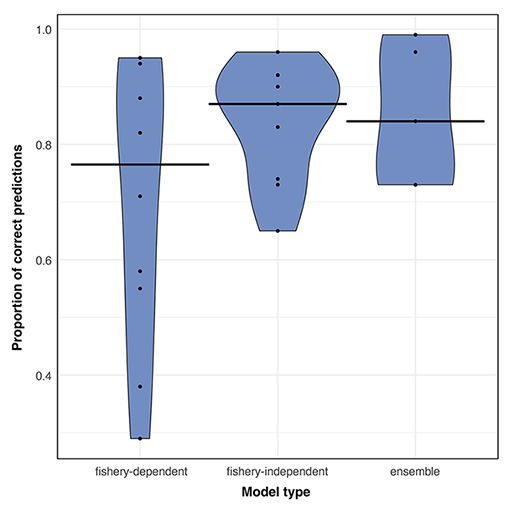
Our results highlight important inherent differences among models built with different types of ecological data. Our results also suggest an integrated approach that combines fishery-dependent and -independent data in a model ensemble is needed to leverage the spatial and temporal coverage of fishery-dependent data and the predictive skill of models based on fishery-independent data.
Model ensembles generate robust predictions of how individual species will respond to projected climate changes. Combining results from multiple data sets balances the sampling strengths and weaknesses of different data types and yields the strongest results.